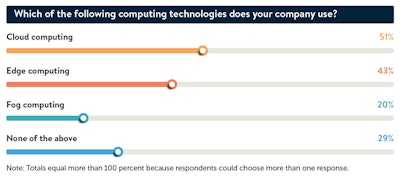
In the world of the Industrial Internet of Things (IIoT), the noisy debate over cloud vs. edge computing masks a key fact: Manufacturers are mapping out a route that covers both, each an integral on-ramp for new predictive maintenance and performance monitoring applications at different stops on the journey.
Almost half of the respondents (43 percent) to a recent Automation World survey already have edge computing implementations underway; just a little more than that (51 percent) have ventured into cloud computing territory as part of their IIoT implementations. Fog computing, considered a superset of edge computing used to bridge the traditional operations (OT) world with enterprise IT, is also starting to take root, cited by only 20 percent of respondents.
More than a third (39 percent) of the manufacturers responding have been using edge/fog or cloud computing technology for one to two years, and 48 percent have been invested in programs for significantly longer (three years or more). Only 12 percent of respondents are just getting started with edge/fog or cloud computing, launching initiatives in the past year or less.
Edge computing was the more popular path for production/manufacturing data analysis applications, cited by more than half of respondents (52 percent), while equipment data analytics—for capacity or overall equipment effectiveness (OEE)—enjoyed slightly less traction with this compute model, cited by just under 20 percent of respondents. Both edge (18 percent) and fog computing (27 percent) were less likely to be the preferred paradigm for enterprise effectiveness analysis compared with the cloud, which was cited by 36.5 percent of respondents.
Regardless of deployment model, manufacturers are already seeing benefits from these initial IIoT deployments. Half of the companies launching edge/fog or cloud computing IIoT initiatives report significant reductions in downtime. At the same time, 38 percent are enjoying measurable improvements to production output, 37 percent tout profitability increases, and 30 percent highlight a decrease in production costs, the survey found.
Though there’s no singular recommended route, IIoT deployments do follow a typical rollout pattern: Phase one is usually centered around the cloud to host core enterprise analytics applications to evaluate plant performance on quality issues, for example, or for asset optimization designed to reduce production downtime. From there, manufacturers invest in new edge computing technologies or retrofit legacy automation systems with intelligence and connectivity horsepower to advance the second phase of deployment, which involves doing detailed analytics in near real time, directly at the point of asset operation. Eventually, the cloud is looped back into the mix as a means of providing additional storage and compute scalability as well as to collect and analyze data beyond a specific industrial asset or single plant floor operation.
“To enable digital transformation, you have to build out the edge computing side and connect it with the cloud—it’s a journey from the edge to the cloud and back, and the cycle keeps continuing,” says Tony Antoun, senior vice president of edge and digital at GE Digital. “You need both to enrich and boost the business and take advantage of different points within this virtual lifecycle.”
A natural progression
Antoun describes three common IIoT deployment scenarios. In one, sensored industrial assets send signals to cloud-based business intelligence tools, dashboards and asset performance management software to track a machine’s health and to trigger basic red- and green-light alerts used to more effectively manage uptime and performance. A second scenario involves these same applications with additional processing capabilities at the edge to trigger an action back at the machine level—for example, opening or closing a valve or resetting an actuator to improve performance or to fix a problem that might cause unnecessary downtime. In the third scenario, edge analytics help avoid unnecessary bandwidth, enhance security and initiate a near-real-time response at the machine level, but the results are also pushed up to the cloud and merged with other data for additional enterprise-level visibility and machine learning-enabled insights.
The choice between edge or cloud computing comes into play depending on what manufacturers are trying to accomplish and where they want to begin. Consider the example of additive manufacturing printers used to build key components for an airplane engine, which Antoun describes as a natural candidate for an edge computing approach. With edge technology, data is collected and analyzed at the point of operation to determine whether the printer is aligned properly prior to production, allowing any necessary adjustments to be made in real time so costly rework is avoided.
A different example, an offshore wind turbine farm with 100 units, showcases where cloud computing is a natural play. In this case, edge processing is tapped to make critical adjustments to individual wind turbines, yet the cloud aggregates signals from the entire fleet and combines it with weather data to support an algorithm that automatically calibrates turbine speeds and blade placement for optimal fleet performance.
“Factories are moving at different speeds, from different angles,” Antoun explains. “Fog and cloud and edge are different approaches, but they don’t negate each other. They actually complement each other at different places in IIoT’s evolution.”
The cloud’s strength for IIoT applications lies in the infinite scalability of compute and storage capacity and in the ease of deploying analytics, given the variety of applications already available. The cloud is also a way for manufacturers with brownfield environments to take a spin with IIoT applications at less expense and disruption, given the longevity of programmable logic controllers (PLCs) and other automation technologies, which simply can’t support edge analytics without a costly and disruptive retrofit, notes Matt Vasey, director of IoT business development at Microsoft and a board member of the OpenFog Consortium, which promotes and standardizes fog computing technologies.
Remote monitoring and predictive maintenance applications are natural targets for cloud deployment because there is no need for a real-time response, explains Jason Andersen, vice president of strategy and business line management for Stratus. “If you’re doing data collection, perhaps sensing temperature to do red light and green light operations, it makes sense to start in the cloud because it’s easier and it’s not something that potentially impacts the production line or business model,” he says. “On the other hand, edge applications tend to be more real-time and mission-critical, so it makes sense that you don’t take on that type of project first.”
Edge computing shines when there’s a need for real-time, latency-free action at the source on a machine or near an operator and when you want to apply the power of machine learning in an industrial context, explains Rhonda Dirvin, another OpenFog Consortium board member and director of IoT and embedded systems at Arm, a semiconductor and software design company. She cited a robotic arm as an example where an edge approach would be favored over cloud deployment. “In a factory environment, latency is an issue—you have to make a decision locally and you don’t have time to send data up to the cloud,” she explains.
Fog creates a new and improved edge
Fog computing is another element of IIoT architecture gaining traction. Though many use fog and edge computing interchangeably, experts see fog as a superset of edge computing, also designed to push intelligence down closer to where data originates. Instead of residing at a device level, fog processing power resides at a local area network (LAN) level, enabling data from multiple assets to be aggregated, filtered, pre-processed and eventually securely transmitted to the cloud using protocols such as MQTT or HTTP.
One benefit to this approach is better security because data is aggregated before transmission to the cloud, so there are fewer pinholes or opportunities for spoofing or data breaches. “We are leveraging the architecture of edge and fog to greatly improve security and to minimize the amount of traffic being pushed to the cloud,” notes Jim Chappell, vice president of information solutions at Schneider Electric, which as part of its merger with Aveva offers a full suite of industrial software. “We want the ability to do everything in the cloud, but edge is a means of optimizing the cloud experience in terms of latency, bandwidth and security.”
Fog computing not only bolsters security and reduces points of failure, but it also bridges the IT/OT divide while breaking the “logjam of PLC purgatory,” explains David King, CEO of FogHorn Systems, which bills its platform as industrial-grade analytics designed specifically for resource-constrained edge devices like PLCs, gateways and industrial PCs.
“OT has been living in a closed-loop automation world the last several decades while the IT world has largely had infinite computing,” King explains. “As you start to collapse the two together, the purpose of fog computing is to serve as a bridge layer between OT and IT. It’s a higher-order layer of compute power.”
FogHorn’s technology employs complex event processing (CEP) analytics on live data to deliver cloud-like computing capacity at the edge instead of simply using edge intelligence to gather and pre-process data before transmission to the cloud for sophisticated machine learning analytics. “A lot of what we’ve seen from edge players is not really doing anything other than collecting and normalizing data and getting it somewhere else to do the heavy lifting,” King says. “We’ve turned that model on its head—we can offer the same capacity that you would otherwise do in the cloud in a fraction of the footprint right at the source.”
On the hardware side, Hewlett Packard Enterprise (HPE) is also flipping the model of what is typical for edge or fog computing, leveraging its enterprise-class server, memory and storage technologies to create the Edgeline Converged Edge Systems. This family of systems can handle full-scale data center analytics and machine learning processing for IIoT applications at the edge, enabling a foundation for real-time processing that will empower new types of industrial, edge-based applications, says Tom Bradicich, vice president and general manager for HPE’s servers, IoT and converged edge systems.
Even in scenarios where edge computing is capable of data-center-level processing, the dueling routes to IIoT will still work together synergistically, King says. Cloud becomes the global repository for a fleet-wide view of all assets and it is also central to the process of refining and updating models for IIoT-enabled applications. “Even here, it’s not edge vs. cloud, but edge to cloud,” he explains. “We believe [artificial intelligence] in the industrial context means permanent, iterative edge-to-cloud machine learning to automate the process of updating the model.”