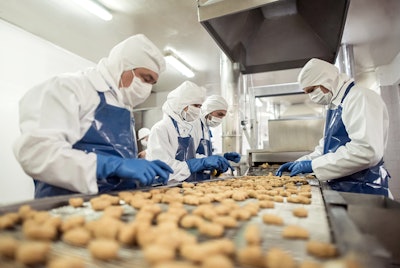
Machine vision has long found a place in food safety, working 24/7 without fatigue. But as data access increases and processing power improves, machine vision is finding even more opportunities through the added capabilities of artificial intelligence (AI).
To take one example, traditional machine vision tends to struggle to inspect for contamination in sun-dried tomatoes. But it’s an application that’s well suited to AI. “Similar to a human, AI is very good at dealing with a lot of variations in whatever’s being looked at,” says Quinn Killough, senior business development manager for Landing AI, a company that provides end-to-end AI platforms for manufacturing. “That type of application, because there’s so much variability in what a tomato could look like or what kind of contamination could be on it, it was a pretty tough machine vision problem in general. A human can do it easily. And it turns out AI can do it fairly easily as well. Being able to deal with all that variation in what you’re looking at, it makes it very well suited for AI.”
As a general rule of thumb, if a human could come up with a decision in their head in less than a second, it’s something that AI could handle. But then why not stick with humans for the task?
See it Live at PACK EXPO Connects Nov. 9-13: Improving Capital Projects Outcomes via Digitial Twins, by Zachry Engineering Corporation. Preview the Showroom Here.
“If you have a human out there inspecting products, they are subject to change in a lot of it. What they think might be a defective piece of food one day they might say is an OK piece of food the next day. They are impacted by a variety of things—they could be in a bad mood that day, or they could’ve forgotten their glasses that day, something like that,” Killough explains. “Machine learning will be able to perform at the best human’s ability consistently, 24/7. There’s no variation in the accuracy with which the machine learning model will look at something and provide a prediction.”
As another example where AI fits well, Killough describes a leak inspection method in which a packaged product is submerged in water with pressure added to the tank to try to pull air out of it. “The human will sit and look for bubbles coming out of this package,” he explains. “With cognitive fatigue, they would eventually miss something, or something might get in the way of their vision and bad products would get through the production line. What we did was create an algorithm that can look at these packages and detect these bubbles consistently and work 24/7.”
Another inspection problem that traditional machine vision has struggled to deal with are skewed date codes on the bottoms of soup cans. “You need to make sure that you have the right date code on that soup tin, but because there’s ridges on the bottom, it gets printed and gets all skewed,” Killough describes. “A traditional system won’t be able to read that, and AI actually does it pretty easily.”
For this, Killough makes a distinction between the typical rules-based machine vision and the machine learning of AI. “The traditional way of doing it is hard coding with rules-based algorithms—what the camera should be looking for,” he says. “It’s very limited in its capabilities because it’s hard coded so it wouldn’t be able to deal with all the variations that I’m talking about in these applications. Whereas machine learning powered machine vision is trained with a ton of images, so it sees all that variation. It learns the variation and learns to kind of accept it.”
Making AI easier
Though AI certainly has its benefits, deploying it can be a challenge if you don’t know what you’re doing, Killough says. “So we tried to tie it all together based on all the machine vision projects that we’ve worked on into this end-to-end platform that essentially streamlined the whole workflow from project inception to deploying a model in production—and taking that deployed model and scaling it out across multiple facilities,” he explains.
Because too often engineers are spending up to 80% of their time just preparing and labeling data, Landing AI has put a lot of its platform’s focus on the data preparation side of things. “We streamlined that process to enable those machine learning engineers to collaborate with the subject matter experts on plant floor that actually know what defects look like and what they don’t look like.”
Landing AI was founded in 2017 by Andrew Ng to help manufacturers move their AI project from proof of concept to full-scale production. Having worked in large tech companies like Google and Baidu, Ng saw the value that AI could bring to those with the data and technical competence to handle it. “But what he realized was that there’s this long tail of other potential benefiters of AI that maybe don’t have the technical expertise or the data to be able to create AI solutions and get the value out of it,” Killough says. “So the idea behind Landing AI is really to enable that long tail of users to get the value out of AI.”
That’s a profile that fits a lot of companies in the manufacturing space, where machine vision tends to be a high-value solution, Killough notes. “We’ve seen a whole variety of adoption levels within customers,” he says. “There’s ones where machine vision is already pervasive but they still have problems that they need help solving. There’s ones that know that they could benefit from machine vision, but don’t really know how to tackle it. And then there’s ones that haven’t really considered it, but they definitely can benefit from it.”
Landing AI tends to interact most with that middle group—those that know that they can benefit but don’t really know where to start.
See it Live at PACK EXPO Connects Nov. 9-13: Affordable shrink sleeve labeling provides product appeal and security, by American Film & Machinery. Preview the Showroom Here.
Pandemic push
With the COVID-19 pandemic making it more difficult for food producers to operate with their usual workforce levels, there’s been an even bigger push into discovering the capabilities of AI. The guidelines released recently by the Centers for Disease Control and Prevention (CDC) for meat and poultry processing workers—including distanced workers and physical barriers—is just one example of how the food industry is expected to conduct its operations amid the pandemic.
The situation is leading in part to the increased use of robotics, but it’s also leading to more interest in AI. “We’ve certainly seen some additional interest for that reason,” Killough says, noting recent interest from key chicken producers. “They realize how vulnerable they are to situations like this. And now they’re thinking to the future of when this might happen again. Or just in the present case, how long this is going to last currently. Automation really helps with putting them to ease with some of those concerns.”
Landing AI has been surprised at how advanced some of these companies are on their AI journeys, according to Leo Zou, director of communications for Landing AI. “We also learned some of the difficult challenges they face when integrating or managing their AI solutions, which leads to the work we do,” he says.
Trying to get a better sense of where manufacturers fall on the spectrum of integrating AI into their visual inspection systems, Landing AI is in the midst of a joint research study on the topic with the Association for Advancing Automation (A3). “We wanted to really get a sense of where the companies are,” Zou says. “Some of them are more advanced, some of them still haven’t even started, some of them are figuring it out.”
PACK EXPO Connects – November 9-13. Now more than ever, packaging and processing professionals need solutions for a rapidly changing world, and the power of the PACK EXPO brand delivers the decision-makers you need to reach. Attendee registration is open now.