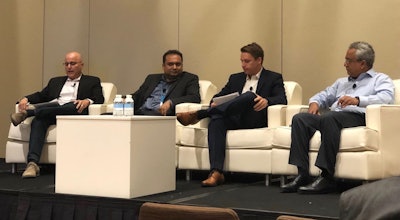
When it comes to the Industrial Internet of Things and Big Data, there are currently two camps out there: The people who are 100 percent committed to adopting the technology and the people who have not bought into the idea—at all. These are two opposite ends of the spectrum, but there is room in the middle of these extremes for those manufacturers that want to dabble in the data without taking a big business risk.
That was the message from three experts on the subject who sat on a panel moderated by Automation World’s Editor-in-Chief David Greenfield at the Siemens Automation Summit this week.
The panel started by addressing the misconceptions that surround the topic, which range from data quality over quantity to overall expectations.
“There’s a big misconception that [manufacturers] will see results tomorrow,” said Jagannath Rao, senior vice president of IoT strategy for Siemens PLM Software, explaining that companies often enter into an IoT and Big Data project assuming their business will change immediately.
“Predictive maintenance is hype,” Pal said. “Machine lifestyle is what matters.” Pal likens this concept to the way in which we take care of ourselves to avoid sickness and disease. For people, lifestyle starts with unadulterated food and clean water. Machines are fed by electrical power, and they too need quality power. Electrical lines can be adulterated with high levels of harmonics, phase imbalance and ground noise. Each of these can kill the machine’s motor.
“If you don’t care about that, then the machine will fail consistently,” Pal said. “I suggest [manufacturers] start to focus on understanding the lifestyle of their machines. It’s as simple as changing the oil and feeding them the right kind of power.”
The other area that creates confusion during these projects is understanding the kind of data that is being collected. “There’s a huge rush to grab a bunch of data, but the focus should be on the quality of the data to make sure everything you are bringing into an IoT system has been carefully selected,” said Andrew Crowley, a system engineer at DMC Inc., a software and engineering services company that offers and integrates manufacturing automation and intelligence technology.
Once a manufacturing team gets beyond the hype and begins to understand the basics of data collection and machine lifestyle, Greenfield asked the panel: “How do you get started with IoT and Big Data to make sure you are doing it right? What are the firsts steps to focus on?”
First and foremost, the group concurred, is to get executive sponsorship for what is sure to be a long journey. Then, the next tangible step includes identifying the key data points and assets that will provide the greatest return on investment. “The initial data points will be tough, so make sure you are willing to work for them,” Crowley said.
Second, make sure there is a scalability strategy because “at the enterprise level it is not machine data alone that makes the difference,” Rao said. There are so many different data sources, from SCADA to CMMS to MES, that generate information. “You need a broader range of data to get more efficient about root cause [analysis]. And, the more connected data sources, the more need to scale repositories, because you won’t do that on premise. You need to scale compute power.”
Pal agreed. “Consider the cost and scalability of your infrastructure,” he said.
In addition, think about what technologies will be used, as there will be Ethernet and TCP/IP interfaces, as well as cybersecurity software. To that end, make sure to involve OEMs in the conversation and even acquire the source code that’s being delivered with the machine, if possible, so that there is access to higher level controls to pinpoint what is being monitored vs. looking down at the sensor level.
Once you’ve connected data sources and are able to get data, the next step is to understand how to get better yields from the assets. But, again, this won’t happen overnight. “It is a journey that can take 12 to 18 months before you get comfortable,” Rao said.